FDA-led consortium studies advance quality control of targeted next generation sequencing assays for precision oncology
Introduction
As precision oncology evolves, understanding and anticipating challenges will be essential to ensure continued forward progress. Targeting cancer with much greater therapeutic accuracy is the aim of precision medicine (oncology) (1). Biomedical advances have converged in the rapidly evolving field of precision oncology with a proliferation of complex tests aimed at identifying biological indicators (biomarkers) on an individual basis from a patient’s tumor sample. Precision oncology requires getting the biomarker tests “right” in order to individually customize treatment, improve outcomes, and at the same time advance our understanding of the role of genetics in cancer (2). A bad biomarker test is as problematic as a bad drug, so getting the biomarker test right is critical (3).
The recent rate of advancements in precision oncology has been staggering. Approximately ten years ago the FDA and NIH announced a shared vision of personalized medicine along with the scientific and regulatory structure needed to support its growth (4). Three years later they jointly announced the policy approval to allow next generation sequencing (NGS) as a platform technology to be used for the development and running of innumerable new genome-based tests with clinical implications and indications (5). President Obama’s 2015 precision medicine initiative expressed strong conviction that science offers great potential for improving health, and oncology was the clear choice for enhancing the near-term impact of this endeavor (6).
Today, there are a number of FDA approved NGS-based solid tumor tests serving as companion diagnostics. These tests provide essential information for the safe and effective use of a particular drug as part of an individualized therapeutic plan for a cancer patient (7,8). A few examples include multiple tumor profiling tests, for instance: FoundationOne CDx, MSK-IMPACT, Oncomine Dx Target Test, Omics Core, and PGDx elio tissue complete. NGS-based tests can also be used to detect DNA shed by the tumor into the blood, termed a liquid biopsy. Most recently, FDA has approved tests for the monitoring of advanced disease using liquid biopsies, e.g., Guardant360® CDx (9), and FoundationOne® Liquid CDx (10).
Unmet needs to advance precision medicine include the development of best practices, protocols, and quality metrics for NGS-based diagnostic assays. The Sequencing Quality Control Phase 2 (SEQC2) consortium was formed to address these needs. SEQC2 is an international group composed of members from academia, government, and industry, and led by the U.S. Food and Drug Administration (FDA) (11). SEQC2 builds on the foundation and frameworks put forth by the Human Genome Project and the many studies from The Cancer Genome Atlas (TCGA) (12,13). SEQC2 is funded by the United States Congressional 21st Century Cures Act (14) and is intended to support cancer research, including the development of more sensitive diagnostic tests and research with the potential to transform the oncologic scientific field.
In this paper, we profile the most recent accomplishments of the SEQC2 Oncopanel Sequencing Working Group. In brief, we have developed a translational scientific infrastructure and applied it to common precision oncology situations. This includes the design and construction of a comprehensive and robust set of nucleic acid (DNA, RNA) reference standards (15) that were used in related SEQC2 studies (Figure 1), which will become publicly available. These related studies include: (I) comprehensive solid tumor onco-panel testing (16); (II) liquid biopsy testing (17); (III) testing involving formalin fixed paraffin embedded (FFPE) materials (18) and; (IV) testing involving spike-in materials (19). All these studies detail the bioinformatics frameworks used and importantly provide best practice recommendations. We also provide a vision for current and future SEQC2 efforts. These efforts aim to assist with the on-going evolution of precision oncology.
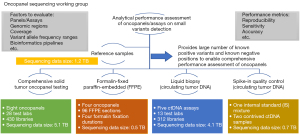
Findings from SEQC2 Oncopanel Sequencing Working Group studies
Establishing a reference sample with known variants
To evaluate the analytical validity of a targeted sequencing panel, a new reference sample had to be created. All other available reference samples either had variants with too high of an allele frequency [population genetics reference samples (20)], too few variants total [based on a single cell line (21-23)], or a limited source (from a tumor). To overcome these three limitations, the SEQC2 working group pooled genomic DNA extracted from 10 Universal Human Reference RNA (UHRR, Agilent Technologies, Inc., Santa Clara, California, USA) cell lines (Figure 2) to make a reference sample (15). This mixture of cancer samples increased the mutation density per gene, which is ideal for being able to assess oncopanel performance across conditions. Sample A was then diluted into a normal sample with known germline variants (Sample B) at various ratios to create a panel of lower allele frequency of variants, and thus more faithfully represent the reality of a tumor sample or liquid biopsy. These samples are publicly available through Agilent Technologies.
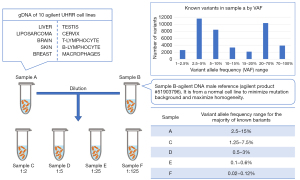
To confidently establish a large number of known positive variants and negative positions, four different whole exome sequencing (WES) enrichment kits and one whole genome sequencing (WGS) method were employed to sequence Sample A and gDNA samples from each cell line individually. These approaches included both short and linked read technologies. By leveraging this wealth of data, a consensus targeted region (CTR), spanning about 22 Mb, was established. The CTR was defined as the coding regions of the intersection of the four WES panels against the NIST high confidence regions, after removal of low complexity regions. Meeting the goal of establishing a sample with a rich collection of mutations, over 40,000 variants down to 1% variant allele frequency (VAF) were identified in the reference Sample A CTR. Meeting yet another goal, more than 25,000 of the variants had VAF of less than 20%, including 1,653 variants in 723 cancer genes listed by the Catalogue Of Somatic Mutations In Cancer (COSMIC) cancer gene census project (24). Over 10 million negative positions (i.e., positions of reference alleles) were identified in Sample A. While known variants can be used to evaluate sensitivity of oncopanels, this enormous collection of known negatives will enable accurate measurements of an oncopanel’s false positive rate. An orthogonal technology (digital droplet PCR) was used to validate a large number of known positive variants and negative positions, serving to confirm this reference sample.
The establishment of this reference sample was key to enabling assessment of oncopanels in a context truer to the clinical setting. The mixture of Sample A and Sample B with different ratios allowed for titration of the known variants with lower VAF (for example, around 0.1% in Sample E), a range suitable for assessing liquid biopsy panels. The broad and deep collection of variants spanning key driver genes at various frequencies enabled assessments of tumor relevant oncopanels in the context of rare subclonal mutations. The group has made the set of known variants and negative positions openly available (15) to the community, enabling others to interrogate additional approaches to targeted oncopanel somatic mutation detection. Lastly, the study serves as a guide to others who may want to establish a reference sample to answer related but different questions.
Interrogating best practices for oncopanel somatic variant detection
To assess best practices of deep targeted sequencing using oncopanels for accurately detecting genetic variants, the group performed a cross-platform multi-lab evaluation of eight panels relying on the variants identified from Sample A. Samples A, B, C and a spike-in sample of synthetic hotspot mutations were tested with four technical replicates for each sample across three independent laboratories for each oncopanel. Using known positives across a range of VAFs and in selected important genomic regions, this study queried both intra-lab and cross-lab reproducibility, known positive detection sensitivity and false positive rate. Given the increasing prevalence of tumor mutational burden (TMB), being leveraged to immune checkpoint inhibitors success (25,26), the group specifically queried the ability of oncopanels to evaluate TMB. Impacts of panel size, reproducibility, and false positive rate on TMB measurements were studied to derive recommendations for using oncopanels to evaluate TMB.
High sensitivity was obtained for all panels across the CTR and all variant types. The overall sensitivity was high across VAF ranges in all panels. It ranged from 87.1% to 98.3% for the lowest VAF range (1–2.5%). A review of all results taken together revealed that a hard VAF threshold can successfully control the false positive rate. With the default VAF threshold of each panel, FP rates were lower than 10.5 per Mb. The majority of FP calls were in the low VAF ranges (<5%). But at the cost of reduced sensitivity due to the inherent variability in VAF measurements. Better overall performance in terms of reproducibility and false positive rate was achieved by restricting variant detection to only the CTR. Reproducibility varied across panels and usually was over 95%. False positives appeared much more commonly outside CTR; thus reproducibility was more challenging outside of this area. For TMB estimation with clinically relevant parameters, the authors recommended that the analysis be restricted to the CTR, the panel target at least 1 Mb within the CTR and a VAF cutoff of 5% be applied. Together with the adequate reference samples developed by this working group, this comprehensive study provided actionable guidelines for oncopanel sequencing and documented clear evidence supporting a simplified and straightforward workflow to assess the analytical performance of oncopanels.
Fixation time- and within block position-dependent quality degradation in FFPE processed samples
The growing trend of minimally invasive surgery (27) means that surgically collected tissue specimens are more limited in size; thus are FFPE processed to meet the many current standard care needs (28). FFPE processing can have considerable consequences for the analytical utility of downstream NGS assays, as the FFPE process induces substantial and highly variable effects on nucleic acid quality and quantity (29). In this study, adopting an FFPE procedure highly analogous to cell block cytology sample processing, the SEQC2 Oncopanel Sequencing Working Group made multiple FFPE blocks of Sample B cell culture at four different formalin fixation duration, 1, 2, 6, 24 hours. Thus, the findings from this study would be readily applicable to clinical FFPE samples. The set of known variants in Sample B were then leveraged to investigate the influence of FFPE processing on reproducibility and accuracy of mutation detection per different oncopanels, formalin fixation duration, and locations (18). As the comprehensive cross-lab oncopanel study confirmed high sensitivity in variant detection, the investigation here was centered on false positive rate, i.e., the frequency of false positive variant calls. Additionally, achieving a low false positive rate is essential for accurate TMB measurements.
Rigorous analysis revealed that cell count was an essential measurement for the quality of sequential FFPE sample sections. A low cell count always leads to a high false positive rate. Another key driver was DNA input amount. Slightly lower DNA input leads to a substantially increased false positive rate. A lower quantity of high-quality sample was observed in samples with longer formalin fixation times. Interestingly, physical position within the FFPE block also had an impact on quality. This study recommended avoiding performing NGS assays on surface FFPE samples, instead selecting samples taken from the core of the block. To get the most from an FFPE sample, the study recommended high cell count, high DNA input amount, minimal formalin fixation duration, and a sample taken from the middle of the block.
Maximizing the analytical validity of circulating tumor DNA sequencing assays
Although clinical adoption of liquid biopsies is currently increasing, results from orthogonal approaches or technologies do not always agree (30-32). Moreover, shared effort between ASCO (American Society of Clinical Oncology) and CAP (College of American Pathologists) detailed the urgency of employing reference samples to compare the analytical validity of circulating tumor DNA (ctDNA) assays across technologies and laboratories and enabling unbiased comparisons across technologies and laboratories (33). The SEQC2 Oncopanel Sequencing Working Group tested five ctDNA assays across 12 laboratories, leveraging a simulated sequencing dataset, a synthetic DNA spike-in dataset and the carefully studied reference sample (17). For this specific study, Sample A (cancer sample) and Sample B (normal sample) were mixed with 20% A/80% B and 4% A/96% B to create a deep collection of variants at many different VAF ranges.
Taking all results together, the authors described the importance of a VAF threshold of 0.5%. Above this limit, mutations were detected with high sensitivity, precision, and reproducibility by all tested assays. Input material amount became a key driver of success or failure below 0.5% VAF. Strikingly, below 0.5% VAF there was a bias toward false negatives rather than false positives. This suggests that rare ctDNA fragments may fall victim to stochastic sampling and simply not be present if the input material is too small and the frequency is too low. In addition, a review of results showed that fragment depth was a driving force for successful detection of low-frequency mutations. For those conducting ctDNA assays, the authors suggest focusing on efficiency and stability of capture enrichment, NGS library conversion and amplification to ensure sufficient and more uniform fragment depth. When studying the impact of unique molecular identifiers (UMIs), results showed that UMIs were indeed helpful for consensus error correction. The use of UMIs is suggested in cases where it is desired to reduce false positive detection.
Spike-in materials to advance quality-control for circulating tumor DNA NGS mutation detection
For clinical liquid biopsies, a limit of detection (LOD) is established in advance for each mutation type or specific mutation, and then that limit is applied equally to every sample. However, previous studies have clearly demonstrated the existence of both variant-specific and sample specific LOD (34). Thus, certain variants with lower VAFs could potentially be measured in certain samples. A second problem with a pre-established LOD is sample or lab-based technical artifacts (35,36) and/or stochastic issues connected to small quantity or poor quality samples (37,38). Taken together, these challenges presented the need to develop methods able to pinpoint sample- and variant-specific LOD. The SEQC2 group designed a synthetic internal standard spike-in for each actionable mutation target suitable for use in NGS following hybrid-capture enrichment and UMI or non-UMI library preparation. To appropriately study the results, the authors developed computational methods to distinguish the spike-in internal standard (IS) controls from the sample native template (NT) sequence reads in FASTQ files prior to pipeline analysis and variant calling. By measuring the error rate at each actionable mutation target through sequencing reads of the spike-in controls, the authors developed a complete set of bioinformatics methods to improve quality control for ctDNA mutation detection.
The authors first assessed whether the spike-in would impact vendor variant calling performance and did not observe any impact. Strikingly, the variant calling in the SEQC2 contrived reference ctDNA was improved using the synthetic spike-in. Digging into the results, the authors noted improved sensitivity without loss of specificity. Importantly, the inclusion of the spike-in enabled calculation of a per variant, per nucleotide, per sample LOD. Other key molecular diagnostic testing methods already leverage synthetic spike-ins as controls (39-41). The work presented by the authors enables extension of this level of quality control to NGS diagnosis of actionable mutations.
Summary and ongoing studies
Sequencing of tumor tissue or blood from a patient can detect driver mutations, but only down to a certain mutation frequency. Low frequency mutations can represent subclones within the tumor, or the presence or absence of DNA shed by a tumor in serum. To boost detection power of these low frequency variants, sequencing reads are focused in areas likely to harbor important driver mutations to boost resolution. These targeted sequencing approaches were subjected to various challenges, including low mutation frequency, FFPE processing, and a liquid biopsy context. To perform the above rigorous testing, the SEQC2 Oncopanel Sequencing Working Group created a set of reference samples and robustly established a list of known variants and negative loci. Various technical factors, lab factors, bioinformatics approaches, genomic context, and the use of spike-in controls were assessed to establish recommendations for best practices. These studies also established false positive rate, calculated as the number of false positive variant calls per million bases of a targeted genomic region, as a key performance metric to evaluate the impacts of underpinning factors including those listed above. Thus, the reference samples and our performance assessment frameworks will enable proficiency testing and routine performance monitoring of oncopanels beyond establishing analytical validity.
Rather than creating a “hit and run” resource, the SEQC2 Oncopanel Sequencing Working Group continues to expand these community resources and more deeply interrogate best practices so that recommendations remain up to date. The current set of known positives is being expanded into more kinds of variants (more indels and multiple nucleotide variants) as well as more areas of the genome (coding regions beyond the CTR). As new bioinformatics methods arise, SEQC2 is working with the community to evaluate them and promote the development of the most accurate analytical approach. For analytical validity, tight and rigorous control of the false positive rate is critical. Thus, SEQC2 continues to dive even deeper into factors impacting the false positive rate, as well as methods to best control the false positive rate. While all data and code from SEQC2 is publicly available, the consortium is working to create a data portal to enable easy access to datasets that can be used to advance the validation and proficiency testing of oncopanels.
Perspectives
Accurate and robust biomarkers are essential for precision oncology. Embodying the convergence of molecular biology, advanced computation (bioinformatics, machine learning and artificial intelligence), data science, and biotechnology, deep targeted sequencing is capable of accurately detecting cancer mutations of low allele frequency; thus enabling unprecedented basic science insights and accelerating the evolution of precision oncology. However, transparent regulation and performance assessment of deep targeted sequencing panels, i.e., oncopanels, was completely unheard of as there was an obvious void of adequate reference samples to support such assessments of analytical performance. Recognizing the urgent need in the field, the SEQC2 Oncopanel Sequencing Working Group undertook a strenuous effort to develop a reference sample harboring a high-density array of small variants at low VAF. Of over 42,000 known variants, over 10 million negative positions were determined within about 22 Mb high confidence coding regions in the human genome. Sample A can then be diluted by Sample B, derived from a normal cell line, to create a set of samples that adjust the allele frequency of those variants to any interested ranges. These high-confidence known variants and known negatives enable the performance assessments such as sensitivity, reproducibility, and false positive rate for individual oncopanels and cross-panel comparison. Adopting the performance analysis workflow for the comprehensive solid tumor oncopanel study, the publicly available reference samples with ample supply can be used to fine tune the experiment protocols, variant reporting regions, bioinformatics pipelines, and VAF reporting thresholds to maximize sensitivity for detecting clinically actionable mutations and control the false positive rate for high accuracy over the entire reporting regions. The false positive rate has a direct impact on the measurement of TMB. After establishing analytical validity, the reference samples can then be used for training and proficiency monitoring of operators, reagents, and instruments.
Liquid biopsy of ctDNA is a key accelerator for the advancement of precision oncology. Five years ago, the application of ctDNA testing in metastatic lung cancer had many concerns. For instance, are liquid biopsy tests sensitive enough for use in a defined clinical setting (cancer metastasis)? How should a negative ctDNA test be confirmed? Is the analytical validity of ctDNA testing in metastatic settings sufficient for clinical deployment? How do critical pre-analytical variables (blood collection tubes and associated processing, molecular extraction of nucleic acids, etc.) affect the performance of ctDNA tests? What are the best-practice workflows (sample collection, sample prep, NGS instrumentation, bioinformatics) for optimal clinical performance and ultimately physician decision making (33)?
In June 2016, FDA approved the first liquid biopsy test, which is PCR-based (42). In 2020 there were two FDA approvals for NGS-based liquid biopsy tests (9,10). Now the strengths of ctDNA testing are gathering attention related to: (I) a more rapid turnaround time vs solid tumor biopsy; (II) ability to assess the primary tumor and metastatic foci (tumor heterogeneity) using a single routine blood draw; (III) safety profile due to the non-invasive nature; (IV) opportunities for longitudinal testing and temporal analyses; and (V) the accessibility of bio-liquids enables new treatment monitoring strategies and patient compliance, as learned from the COVID pandemic. For instance, a phlebotomist can travel to a patient’s home and collect a blood sample for ctDNA analysis. The physician can then adjust the therapeutic regimen. This whole process can occur entirely outside of a hospital.
Figure 3 displays how ctDNA assays can be utilized to guide medical decision making. The x-axis represents time and is segmented by states or phases a patient may have during their treatment course. The y-axis shows the concentration of ctDNA in the blood. Illustrated is the pattern of tumor growth over time measured by ctDNA found in the blood. This is shown following diagnosis (Dx), initial treatment, response to treatment and, relapse with the acquisition of resistance mutations. Currently, the state/phase of ctDNA-based clinical applications are located on the right most portion of the diagram (shaded green), principally under “Metastasis & Relapse”, since there are FDA approvals for these indications. Given the results of the SEQC2 liquid biopsy proficiency study (17) and further maturation of ctDNA assay development, there are opportunities to shift applications of ctDNA targeted to earlier cancer diagnosis (orange shaded). Using ctDNA as part of the consideration for adjuvant therapy, surveillance for cancer recurrence, and more effective Minimal Molecular Residual Disease (MMRD) strategies, will allow for deeper and more durable remissions. Guidance to avoid “over treatment” of patients and reductions of severe toxicities and treatment related malignancies are critical needs that liquid biopsies may help to address.
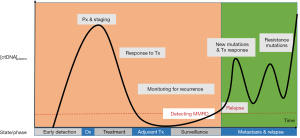
However, there are new challenges in the field of precision oncology. Clonal hematopoiesis of an indeterminate potential (CHIP) is a newly discovered biological process, and affects any type of assay utilizing nucleic acids derived from blood (43). Accurate identification of tumor-derived somatic variants in circulating free DNA (cfDNA) or white blood cells (germline constituent analysis) requires an understanding of the biological sources and processes contributing to the greater source of blood-based mutations (44). CHIP is defined by the presence of acquired mutations in hematopoietic progenitor cells and is age dependent. It occurs in up to 30% of individuals 60–70 years old and in less than 1% of those age 40 or younger (45). The clinical significance of CHIP warrants further study and must be accounted for when interpreting liquid biopsy assays (46,47) or an onco-panel with a matched germline (48).
There are also limitations due to a lack of signal from somatic variant-based assay approaches for early detection, MMRD and screening applications. A major finding from the SEQC2 liquid biopsy proficiency testing study (17) is that the degree of DNA input material impacts test sensitivity, and more input improves sensitivity and reproducibility for variants with VAF below 0.5%. Plasma from healthy donors typically yields ~5–10 ng cfDNA per mL (49). The amount of ctDNA obtained from cancer patient depends on the organ of origin, burden of disease and shedding characteristics of the tumor (50,51). There are concerning limitations for somatic variant-based early cancer detection testing approaches due to sensitivity (lack of signal) and specificity due to non-tumor sources of biological noise (CHIP) causing false positive findings and lowering specificity. Alternative approaches are needed.
Using methylation signatures in cfDNA characterized by machine learning classifiers, sensitive and specific multi-cancer detection (MCD) and tissue of origin localization are now being developed as a next generation liquid biopsy assay (52). The advantages of methylation detection for more sensitive cancer assays are: (I) improved sensitivity since alterations in methylation in the genome provide high sensitivity for cancer detection; (II) improved specificity since methylation pattern identification is not affected by CHIP so this eliminates addressing CHIP-based filtering; (III) tissue of origin can be determined by methylation patterns; and (IV) improved cost effectiveness since the sequencing depth requirements for methylation analysis is much lower compared to cancer-based somatic variant analysis (44). The MCD would work in conjunction with other tests and established screening modalities, for example, if the MCD were positive for colorectal cancer then additional screening (i.e., colonoscopy) would be indicated.
Other representative examples of alternative approaches to improve sensitivity with low amounts of input cfDNA follow. The premise of MRDetect (53) is to use machine learning to predict tumor fractions present in ctDNA as a function of the information obtained from solid tumor samples, specifically mutational load, sequencing depth, copy number alterations and the number of somatic mutations. Using this information, an in-silico plasma mixture model is constructed and used to search WGS from the ctDNA sample in a quantitative and dynamic manner as samples are obtained over time. This approach serves to evaluate changes in disease burden based on sequencing breadth vs. depth.
DELFI (DNA EvaLuation of Fragments of early Interception) (54) uses fragmentation patterns present in cfDNA to distinguish normal vs. cancer, and for cancer indicates the likely tissue of origin. A machine learning model was developed that incorporated genome-wide fragmentation features distinguishing normal fragmentation patterns from cancerous patterns and associated tissue(s) of origin. Two additional ctDNA assays are focused on early-stage colorectal cancer and utilize advanced NGS and analytics (55,56).
A summary of completed and planned projects is provided in Table 1. Due to a lack of DNA and RNA reference materials for NGS-based testing scenarios, SEQC2 investigators put forth the development and construction of robust reference materials used in numerous studies (as reported). Due to the evolving precision oncology efforts utilizing methylation approaches for cancer screening and MMRD, enhanced reference materials supporting methylation assays are needed. Regarding oncopanel testing (as reported), the DNA-based approach is complete and the RNA-based approaches involving expressed mutations and fusions are in progress. FFPE processing is an important and prevalent aspect of clinical samples. The SEQC2 DNA-based FFPE study is complete. However, the growing importance of fixed tissue compounded by increasing relevance of transcriptomics precipitates the need for FFPE RNA-based analysis. The liquid biopsy study is complete and focused on DNA somatic variant analysis for the purpose of analytical validation and proficiency testing. More advanced liquid biopsy applications focused on MMRD and cancer screening involving MCD and tissue of origin are rapidly progressing. These are based on new scientific approaches such as methylation analysis and the use of innovative algorithms employing machine learning. Both enhanced reference samples with methylation capabilities, guidance, and best practice recommendations for establishing machine learning classifiers for clinical assays, analytical validation and proficiency testing for cancer screening and MMRD are needed.
Table 1
Projects completed | Application focus | Projects in progress or needed | Application focus |
---|---|---|---|
Constructing reference materials for the purpose of DNA and RNA analytical validation and proficiency testing | Monitoring pts with an established Dx | Constructing reference materials for epigenetic studies, especially DNA methylation | CA screening; MMRD |
Oncopanel utilizing DNA-based analysis | Monitoring pts with an established Dx | Oncopanel, RNA expressed mutations and fusions (in progress) | Monitoring pts with an established Dx |
FFPE utilizing DNA-based analysis | Monitoring pts with an established Dx | FFPE, RNA-based analysis | Monitoring pts with an established Dx |
Liquid biopsy employing DNA somatic variant analysis for the purpose of analytical validation and proficiency testing | Monitoring pts with an established Dx | Liquid biopsy, methylation-based analyses for multi-cancer early detection (MCED) and tissue of origin (TOO) | CA screening; MMRD |
CA, cancer; Dx, diagnosis; FFPE, formalin fixed paraffin embedded; MMRD, Minimal Molecular Residual Disease; pts, patients.
In conclusion, these SEQC2 studies have developed and made publicly available robust reference sample materials, detailed the bioinformatics frameworks for analytical performance assessments, and provided best practice recommendations. We have also provided a vision for current and future SEQC2 efforts based on the perceived needs in this dynamic space. SEQC2 consortium efforts aim to assist with the on-going evolution of precision oncology best practices, regulatory science, and delivering improved cancer outcomes for patients.
Acknowledgments
Funding: BAA grant HHSF223201510172C (to DJJ).
Footnote
Provenance and Peer Review: This article was commissioned by the Guest Editors (Zhi-Ming Shao and Xiao-Mao Guo) for the series “Eastern and Western Perspectives on Precision Cancer Medicine” published in Precision Cancer Medicine. The article has undergone external peer review.
Peer Review File: Available at: https://dx.doi.org/10.21037/pcm-21-29
Conflicts of Interest: All authors have completed the ICMJE uniform disclosure form (available at https://dx.doi.org/10.21037/pcm-21-29). The series “Eastern and Western Perspectives on Precision Cancer Medicine” was commissioned by the editorial office without any funding or sponsorship. RK is an employee of Immuneering Corporation. Immuneering Corporation has supported her involvement in this manuscript by allowing her to spend company time on it. In addition, Immuneering supports her to attend conferences where she learns information related to the manuscript topic (such as AACR, SOT, AAPS, MAQC Society, etc.). She is also the deputy chair of the MAQC Society (their annual conference and webinar has highlighted SEQC2 speakers). WT is a full time employee of the U.S. Food and Drug Administration (FDA) as well as a board member of MCBIOS Society and AR-BIC Consortium. JX is a full time employee of the U.S. Food and Drug Administration (FDA) and the Executive Secretary of the MAQC Society (their annual conference and webinar has highlighted SEQC2 speakers). The authors have no other conflicts of interest to declare.
Disclaimer: The views presented in this article do not necessarily reflect those of the U.S. Food and Drug Administration. Any mention of commercial products is for clarification and is not intended as an endorsement.
Ethical Statement: The authors are accountable for all aspects of the work in ensuring that questions related to the accuracy or integrity of any part of the work are appropriately investigated and resolved.
Open Access Statement: This is an Open Access article distributed in accordance with the Creative Commons Attribution-NonCommercial-NoDerivs 4.0 International License (CC BY-NC-ND 4.0), which permits the non-commercial replication and distribution of the article with the strict proviso that no changes or edits are made and the original work is properly cited (including links to both the formal publication through the relevant DOI and the license). See: https://creativecommons.org/licenses/by-nc-nd/4.0/.
References
- Ashley EA. Towards precision medicine. Nat Rev Genet 2016;17:507-22. [Crossref] [PubMed]
- National Academies of Sciences, Engineering, and Medicine. Biomarker tests for molecularly targeted therapies: key to unlocking precision medicine. 2016.
- Hayes DF. From genome to bedside: are we lost in translation? Breast 2013;22:S22-6. [Crossref] [PubMed]
- Hamburg MA, Collins FS. The path to personalized medicine. N Engl J Med 2010;363:301-4. [Crossref] [PubMed]
- Collins FS, Hamburg MA. First FDA authorization for next-generation sequencer. N Engl J Med 2013;369:2369-71. [Crossref] [PubMed]
- Collins FS, Varmus H. A new initiative on precision medicine. N Engl J Med 2015;372:793-5. [Crossref] [PubMed]
- In Vito Diagnostics - Nucleic Acid Based Tests. 2021. Available online: https://www.fda.gov/medical-devices/in-vitro-diagnostics/nucleic-acid-based-tests. Accessed 6/27/2021 2021.
- List of Cleared or Approved Companion Diagnostic Devices (In Vitro and Imaging Tools). 2021. Available online: https://www.fda.gov/medical-devices/in-vitro-diagnostics/list-cleared-or-approved-companion-diagnostic-devices-in-vitro-and-imaging-tools. Accessed 6/27/21 2021.
- FDA approves first liquid biopsy next-generation sequencing companion diagnostic test. 2020. Available online: https://www.fda.gov/news-events/press-announcements/fda-approves-first-liquid-biopsy-next-generation-sequencing-companion-diagnostic-test.
- FDA approves liquid biopsy NGS companion diagnostic test for multiple cancers and biomarkers. 2021. Available online: https://www.fda.gov/drugs/resources-information-approved-drugs/fda-approves-liquid-biopsy-ngs-companion-diagnostic-test-multiple-cancers-and-biomarkers.
- MicroArray/Sequencing Quality Control (MAQC/SEQC). U.S. Food and Drug Administration. Available online: https://www.fda.gov/science-research/bioinformatics-tools/microarraysequencing-quality-control-maqcseqc#MAQC_IV (2019).
- Lander ES, Linton LM, Birren B, et al. Initial sequencing and analysis of the human genome. Nature 2001;409:860-921. [Crossref] [PubMed]
- Tomczak K, Czerwińska P, Wiznerowicz M. The Cancer Genome Atlas (TCGA): an immeasurable source of knowledge. Contemp Oncol (Pozn) 2015;19:A68-77. [Crossref] [PubMed]
- 21st Century Cures Act. 2016. Available online: https://www.congress.gov/114/bills/hr34/BILLS-114hr34enr.pdf. Accessed 6/30/21 2021.
- Jones W, Gong B, Novoradovskaya N, et al. A verified genomic reference sample for assessing performance of cancer panels detecting small variants of low allele frequency. Genome Biol 2021;22:111. [Crossref] [PubMed]
- Gong B, Li D, Kusko R, et al. Cross-oncopanel study reveals high sensitivity and accuracy with overall analytical performance depending on genomic regions. Genome Biol 2021;22:109. [Crossref] [PubMed]
- Deveson IW, Gong B, Lai K, et al. Evaluating the analytical validity of circulating tumor DNA sequencing assays for precision oncology. Nat Biotechnol 2021;39:1115-28. [Crossref] [PubMed]
- Zhang Y, Blomquist TM, Kusko R, et al. Deep oncopanel sequencing reveals fixation time- and within block position-dependent quality degradation in FFPE processed samples. Cell Genomics 2021;Prep:2021.04.06.438687.
- Willey JC, Morrison TB, Austermiller B, et al. Advancing NGS quality control to enable measurement of actionable mutations in circulating tumor DNA. Cell Reports Methods 2021:100106.
- Zook JM, Catoe D, McDaniel J, et al. Extensive sequencing of seven human genomes to characterize benchmark reference materials. Sci Data 2016;3:160025 [Crossref] [PubMed]
- Suzuki T, Tsukumo Y, Furihata C, et al. Preparation of the standard cell lines for reference mutations in cancer gene-panels by genome editing in HEK 293 T/17 cells. Genes Environ 2020;42:8. [Crossref] [PubMed]
- Craig DW, Nasser S, Corbett R, et al. A somatic reference standard for cancer genome sequencing. Sci Rep 2016;6:24607. [Crossref] [PubMed]
- Kim J, Kim D, Lim JS, et al. The use of technical replication for detection of low-level somatic mutations in next-generation sequencing. Nat Commun 2019;10:1047. [Crossref] [PubMed]
- Sondka Z, Bamford S, Cole CG, et al. The COSMIC Cancer Gene Census: describing genetic dysfunction across all human cancers. Nat Rev Cancer 2018;18:696-705. [Crossref] [PubMed]
- Samstein RM, Lee CH, Shoushtari AN, et al. Tumor mutational load predicts survival after immunotherapy across multiple cancer types. Nat Genet 2019;51:202-6. [Crossref] [PubMed]
- Chalmers ZR, Connelly CF, Fabrizio D, et al. Analysis of 100,000 human cancer genomes reveals the landscape of tumor mutational burden. Genome Med 2017;9:34. [Crossref] [PubMed]
- Minimally Invasive Surgery Market-Global Industry Analysis, Size, Share, Growth, Forecast 2019.
- AJCC Cancer Staging Manual, 8th edition. Springer International Publishing, 2017.
- Do H, Dobrovic A. Sequence artifacts in DNA from formalin-fixed tissues: causes and strategies for minimization. Clin Chem 2015;61:64-71. [Crossref] [PubMed]
- Stetson D, Ahmed A, Xu X, et al. Orthogonal comparison of four plasma NGS tests with tumor suggests technical factors are a major source of assay discordance. JCO Precision Oncology 2019;3:1-9. [Crossref]
- Kuderer NM, Burton KA, Blau S, et al. Comparison of 2 Commercially Available Next-Generation Sequencing Platforms in Oncology. JAMA Oncol 2017;3:996-8. [Crossref] [PubMed]
- Torga G, Pienta KJ. Patient-Paired Sample Congruence Between 2 Commercial Liquid Biopsy Tests. JAMA Oncol 2018;4:868-70. [Crossref] [PubMed]
- Merker JD, Oxnard GR, Compton C, et al. Circulating Tumor DNA Analysis in Patients With Cancer: American Society of Clinical Oncology and College of American Pathologists Joint Review. Arch Pathol Lab Med 2018;142:1242-53. [Crossref] [PubMed]
- Craig DJ, Morrison T, Khuder SA, et al. Technical advance in targeted NGS analysis enables identification of lung cancer risk-associated low frequency TP53, PIK3CA, and BRAF mutations in airway epithelial cells. BMC Cancer 2019;19:1081. [Crossref] [PubMed]
- Lott R, Tunnicliffe J, Sheppard E, et al. Practical guide to specimen handling in surgical pathology. College of American Pathologists 2015:1-52.
- FoundationOne. FoundationOne CDx Technical Information.
- Blomquist T, Crawford EL, Yeo J, et al. Control for stochastic sampling variation and qualitative sequencing error in next generation sequencing. Biomol Detect Quantif 2015;5:30-7. [Crossref] [PubMed]
- Fu GK, Xu W, Wilhelmy J, et al. Molecular indexing enables quantitative targeted RNA sequencing and reveals poor efficiencies in standard library preparations. Proc Natl Acad Sci U S A 2014;111:1891-6. [Crossref] [PubMed]
- Dolan JQ. When Should an Internal Standard Be Used? 2012.
- Takáts Z, Wiseman JM, Cooks RG. Ambient mass spectrometry using desorption electrospray ionization (DESI): instrumentation, mechanisms and applications in forensics, chemistry, and biology. J Mass Spectrom 2005;40:1261-75. [Crossref] [PubMed]
- cobas® EGFR Mutation Test v2 | Roche Molecular Diagnostics. Diagnostics. Available online: https://diagnostics.roche.com/us/en/products/params/cobas-egfr-mutation-test-v2.html
- Malapelle U, Sirera R, Jantus-Lewintre E, et al. Profile of the Roche cobas® EGFR mutation test v2 for non-small cell lung cancer. Expert Rev Mol Diagn 2017;17:209-15. [Crossref] [PubMed]
- Steensma DP, Bejar R, Jaiswal S, et al. Clonal hematopoiesis of indeterminate potential and its distinction from myelodysplastic syndromes. Blood 2015;126:9-16. [Crossref] [PubMed]
- Razavi P, Li BT, Brown DN, et al. High-intensity sequencing reveals the sources of plasma circulating cell-free DNA variants. Nat Med 2019;25:1928-37. [Crossref] [PubMed]
- Heuser M, Thol F, Ganser A. Clonal Hematopoiesis of Indeterminate Potential. Dtsch Arztebl Int 2016;113:317-22. [PubMed]
- Swanton C, Venn O, Aravanis A, et al. Prevalence of clonal hematopoiesis of indeterminate potential (CHIP) measured by an ultra-sensitive sequencing assay: Exploratory analysis of the Circulating Cancer Genome Atlas (CCGA) study. J Clin Oncol 2018;36:12003. [Crossref]
- Hu Y, Ulrich BC, Supplee J, et al. False-Positive Plasma Genotyping Due to Clonal Hematopoiesis. Clin Cancer Res 2018;24:4437-43. [Crossref] [PubMed]
- Coombs CC, Gillis NK, Tan X, et al. Identification of Clonal Hematopoiesis Mutations in Solid Tumor Patients Undergoing Unpaired Next-Generation Sequencing Assays. Clin Cancer Res 2018;24:5918-24. [Crossref] [PubMed]
- Volckmar AL, Sültmann H, Riediger A, et al. A field guide for cancer diagnostics using cell-free DNA: From principles to practice and clinical applications. Genes Chromosomes Cancer 2018;57:123-39. [Crossref] [PubMed]
- Bettegowda C, Sausen M, Leary RJ, et al. Detection of circulating tumor DNA in early- and late-stage human malignancies. Sci Transl Med 2014;6:224ra24 [Crossref] [PubMed]
- Abbosh C, Birkbak NJ, Wilson GA, et al. Phylogenetic ctDNA analysis depicts early-stage lung cancer evolution. Nature 2017;545:446-51. [Crossref] [PubMed]
- Liu MC, Oxnard GR, Klein EA, et al. Sensitive and specific multi-cancer detection and localization using methylation signatures in cell-free DNA. Ann Oncol 2020;31:745-59. [Crossref] [PubMed]
- Zviran A, Schulman RC, Shah M, et al. Genome-wide cell-free DNA mutational integration enables ultra-sensitive cancer monitoring. Nat Med 2020;26:1114-24. [Crossref] [PubMed]
- Cristiano S, Leal A, Phallen J, et al. Genome-wide cell-free DNA fragmentation in patients with cancer. Nature 2019;570:385-9. [Crossref] [PubMed]
- Tie J, Wang Y, Tomasetti C, et al. Circulating tumor DNA analysis detects minimal residual disease and predicts recurrence in patients with stage II colon cancer. Sci Transl Med 2016;8:346ra92 [Crossref] [PubMed]
- Wan N, Weinberg D, Liu TY, et al. Machine learning enables detection of early-stage colorectal cancer by whole-genome sequencing of plasma cell-free DNA. BMC Cancer 2019;19:832. [Crossref] [PubMed]
Cite this article as: Li D, Kusko R, Ning B, Tong W, Johann DJ Jr, Xu J. FDA-led consortium studies advance quality control of targeted next generation sequencing assays for precision oncology. Precis Cancer Med 2021;4:32.